Written by: James Loughlin, Head of Data Analytics
With the introduction of the new Subset Sampling method into IDEA 11 we felt that it would be a good opportunity to discuss all of the sampling methods available to enable users to calculate sample sizes, extract records, as well as in some cases provide the ability to evaluate the results of sampling as part of substantive audit testing.
As per ISA 530, Audit sampling is the use of an audit procedure on a selection of the items within an account balance or class of transactions. The sampling method selected should give each audit unit, whether it be a record or value in a dataset, an equal probability that each audit unit in the sample could be selected. Audit sampling is needed when population sizes are large, since examining the entire population would not be efficient.
IDEA 11 along with a number of Audit specific data analytic capabilities, also offers a number of Statistical Sampling methods which are commonly used to evaluate for validity in a manner which then allows for further evaluation across a population. Including basic methods such as random or systematic methods, IDEA also includes more sophisticated sampling methods, such as Monetary Unit Sampling and Classical Variable Sampling, which are difficult to perform manually.
Random Record Sampling
Random Record Sampling is used to generate a database of random records taken from an input dataset. Random Record Sampling is a common means of sampling and with this method, users simply define the required sample size as well as the range of records from which the sample is to be extracted. Then, using a random number seed, IDEA generates a list of random numbers and selects the appropriate records associated with these numbers.
If users need to extend a sample, this can be achieved by simply entering the original random number seed used in the first sample and the increased sample count whereby IDEA selects the same sample but extends it to a larger number of items.
Systematic Record Sampling
Also referred to as Interval Record Sampling, Systematic Record Sampling is a sampling method which can be used to extract a number of records from a dataset at equal intervals.
IDEA offers two methods of extracting the sample:
- Entering the number of records, in which case IDEA computes the interval size.
- Entering the selection interval, in which case IDEA computes the number of records.
IDEA calculates the above parameters on the number of records in the database and defaults to the first to last records. However, you can extract the sample from a range of records, if required.
Stratified Random Record Sampling
Stratified Random Record Sampling can be used to extract a random sample with a specified number, or percentage of records, from a series of stratified bands.
This Sampling method is used to ensure that at least one transaction is randomly selected from a targeted period or numeric range of high and low values.
Subset Sampling - NEW
New to IDEA 11, the Subset Sampling method is similar to the Stratified Random Record Sampling method but is alternatively based on not only value alone but also on secondary subsets within the sampled dataset. This method is ideal for scenarios where the dataset contains multiple sub-elements that you wish to sample independently. For example, if a General Ledger report contains a transaction type field (such as Accounts Payable, Accounts Receivables, Inventory, etc.)
Monetary Unit Sampling
Monetary Unit Sampling (MUS) is a method used to estimate the amount of potential misstatement within a list of transactions. MUS is a method where the sampled unit is no longer a record but a monetary amount amount. Biased to items with greater monetary value and giving them a proportionally greater chance of being sampled, MUS has the evaluation facility to predict the financial result of any misstatements, but is used generally on datasets where very little misstatement is expected (no more than 5%).
It is a useful method when testing trade debtors or trade creditors as it ensures focus on higher value items but does not overlook small value items.
IDEA’s MUS method is separated into three stages:
- Planning
- Sample Extraction
- Sample Evaluation
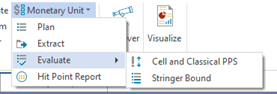
When evaluating your MUS sample(s) IDEA offers two evaluation types:
- Cell and Classical PPS: Used when all your errors are in stored in one file
- Stringer Bound: Used to evaluate samples from multiple files with different intervals at a single confidence level
Every record whose Audited Amount is different to the Book Value is ‘tainted’
Following a distribution formula outlined by a French mathematician Simeon Poisson in 1837, IDEA’s analytics engine adds all the taintings and offers a conclusion based on the parameters that have been entered. Calculation of the Upper error Limit is modified as each tainted docket is processed via a method of Precision Gap Widening.
- Classical Variable Sampling
Classical Variables Sampling is very similar to Monetary Unit Sampling but is generally used on financial data when:
- there is an expectation of moderate to larger number of misstatements, e.g., 5% or more
- either overstatements or understatements may exist
- zero monetary values may exist
Classical Variables Sampling is a method for estimating:
- the total audited value of an account or subset of transactions
- the total amount of monetary misstatement in an account or subset of transactions
Classical variables sampling is unbiased, and it is not based on the amounts contained in a record. Each record has an equal chance of being selected for inclusion in the sample. Note that Classical Variable Sampling will typically generate a larger sample file than other sampling methods.
Attribute Sampling
The final Sampling method within IDEA is Attribute Sampling, which is a method used to assess how frequently a particular event, or attribute, occurs in a given dataset. An attribute has only two values: true or false. In Auditing, typical attributes are whether an item is in error or not, whether a particular control has been exercised or not when it should have been, or whether the entity complied with a particular law or not.
Examples include whether:
- account Receivable balances were overdue or not
- payments were correctly authorised or not
- expense claims were valid or not
Attribute Sampling should be used when:
- there is a need for a statistical sampling solution and judgmental sampling will not suffice.
- the objective of the review is to test compliance to internal controls.
- the compliance testing should evaluate to a true or false result.
- a random selection process will meet the objectives of your review.